You’re collecting data from every direction—online sales, in-store transactions, social media trends—but turning that flood of information into actionable insights?
That’s a different story.
Traditional LLMs rely on static data, leading to irrelevant product recommendations, missed sales opportunities, and inefficient inventory management.
RAG, on the other hand, can combine your data with the latest market trends and customer behaviors, delivering real-time, personalized insights that help you keep pace in your industry.
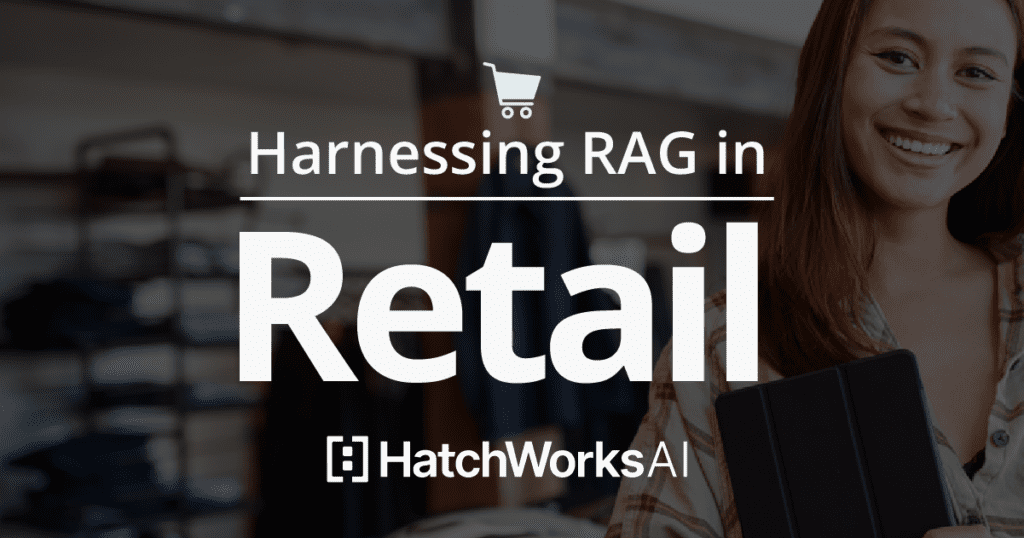
Want to implement RAG right away?
Check out our RAG Accelerator to learn how we can help.
RAG (Retrieval Augmented Generation): A Brief Definition
To get to the bottom of RAG, or Retrieval Augmented Generation, just look at its name. It’s where generative AI, like you see in Large Language Models (LLMs), is enhanced with the ability to retrieve relevant, real-time information.
It then uses that information to generate a response. Sounds simple, right? Here’s what that looks like in terms of architecture:
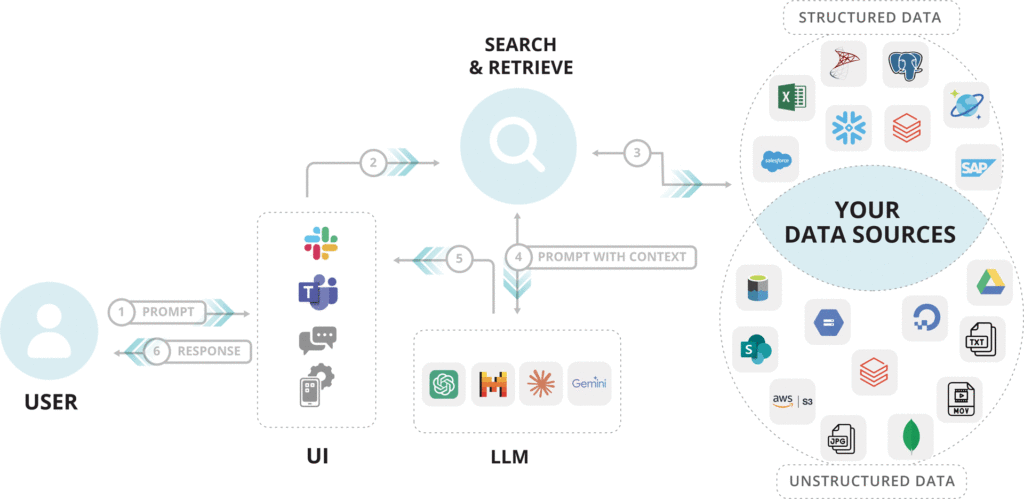
In retail, RAG pulls data from internal sources like customer behavior and inventory, as well as external ones like market trends.
This lets retailers create personalized recommendations and gain real-time insights.
Think of it like an AI-powered assistant that understands your customers, your inventory, and can instantly suggest the right solutions to boost sales and efficiency.
How RAG Differs from Other AI Approaches:
Generative AI and LLMs, rely on static data, generating outputs based solely on patterns learned during training.
In retail, where customer behavior, market trends, and inventory needs change rapidly, this can lead to outdated or irrelevant insights.
RAG vs. Pure Generative Models: Generative AI models produce responses based on historical data, but without real-time updates, they might miss current trends or shifts in consumer demand.
For example, a pure generative model might recommend products based on last season’s sales data, missing out on the current best-sellers.
Whereas RAG dynamically pulls in proprietary data such as customer purchase history, live inventory levels, and seasonal trends, ensuring that every recommendation is up-to-date and context-aware.
Whether it’s adjusting product recommendations based on live stock availability or personalizing offers for returning customers, RAG provides real-time, relevant insights that generative models can’t match on their own.
You can use proprietary data in RAG for:
- Personalization: Tailor product recommendations and marketing campaigns to individual customer behaviors and preferences.
- Competitive advantage: Offer unique insights and products based on your proprietary data that competitors without access to that information cannot replicate.
- Data security: Keep sensitive retail data, such as customer preferences and purchase history, secure within your organization while still using it to power AI-driven insights.
Why Retail Needs RAG: A Summary
With information pouring in from multiple channels—e-commerce platforms, physical stores, social media, and beyond—retailers often struggle to process and use data effectively.
But your customers demand personalized experiences that make purchasing the things they want and need easier or they’ll look elsewhere.
RAG to the rescue. 🦸
With RAG, you can harmonize customer data and create a seamless experience whether someone shops online or in-store. You can even have it suggest products based on past purchases or browsing behavior and adjust recommendations in real time based on inventory levels.
Plus, it keeps sensitive customer data secure, ensuring privacy is maintained while using data to improve customer experience.
That is, if you know how to implement RAG into your business.
At HatchWorks AI, we do—and we do so securely:
Secure and Certified with SOC 2 Type I and HIPAA
At HatchWorks, trust and compliance are paramount. We adhere to the highest security standards, evidenced by our SOC 2 Type I and HIPAA certifications.
These certifications, verified through independent audits, ensure our systems are secure and your data is protected under strict privacy regulations. Stay informed with our real-time TrustCloud compliance program.
Benefits of RAG in the Retail Industry
Let’s look more closely at how RAG can benefit your work in retail.
Personalized Customer Experiences (That Feel Human)
RAG delivers personalized product recommendations, offers, and content by pulling in real-time data like browsing history, past purchases, and even customer preferences.
This makes every interaction feel tailored to the individual.
For example, an e-commerce store can use RAG to suggest products based on what’s trending and in stock, showing customers options they’re likely to buy. The result should be higher conversion rates and a better shopping experience for all.
Differentiation Through Proprietary Data
Retailers collect a wealth of proprietary data that competitors can’t access. With RAG, this data becomes a powerful tool for offering insights and experiences that no one else can.
For instance, RAG can analyze past customer purchases alongside exclusive store data to deliver recommendations that are more personalized and relevant than standard algorithms based on general market trends.
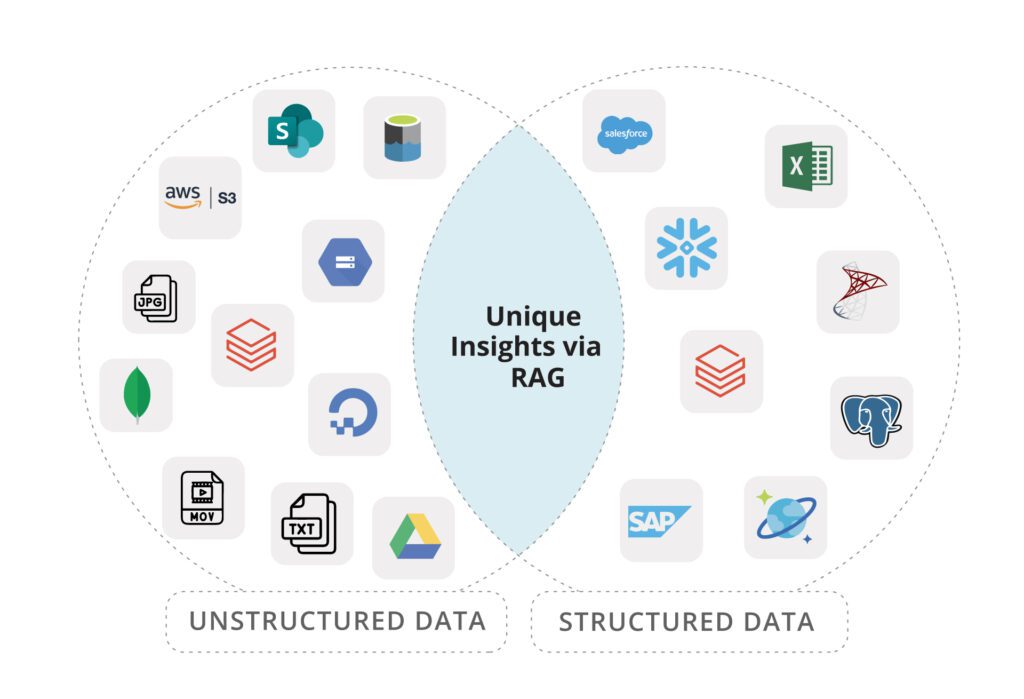
Optimized Inventory Management & Operations
RAG helps retailers manage stock more efficiently by pulling from sales data, customer behavior, and external factors like seasonality. This ensures that shelves are stocked with what customers want, without over-ordering or understocking.
A retailer can use RAG to adjust inventory levels in real time based on what’s selling fast, preventing stock outs or wasted inventory while maximizing sales opportunities.
It can also keep your operations in check and help store workers find out important information fast.
Target is using RAG to help their retail employees work more efficiently. It’s called their “Store Companion” and is meant to streamline store operations and enhance safety and security measures. Users can ask questions about work procedures and get immediate instructions.
Overcoming Challenges
While RAG offers the above benefits, it also presents a few challenges that retailers need to address if they want stress-free implementation and use.Challenge 1: Data Privacy and Security
Retailers handle sensitive customer information across multiple channels, from online transactions to in-store purchases.
With regulations like GDPR and CCPA in place, protecting this data and ensuring compliance is essential to maintaining customer trust and avoiding penalties.
How to overcome it: To address this, retailers should implement secure RAG solutions that encrypt customer data and limit access to authorized users.
Choosing platforms that offer built-in compliance tools and regular audits ensures that your data management aligns with privacy regulations.
You can also hire a company like HatchWorks AI to build a robust RAG solution for you—one where data compliance is built-in from the start.
Challenge 2: Integration with Legacy Systems
Many retailers rely on legacy systems to manage inventory, sales, and customer data. These older systems, while still functional, often lack the flexibility needed to integrate modern AI tools like RAG.
Unfortunately, this can lead to inefficiencies and prevent retailers from fully leveraging their data.
How to overcome it: Start with a pilot project that targets a specific area, like personalized recommendations or inventory management, to test how RAG integrates with existing systems.
Using APIs or middleware solutions can help bridge the gap, allowing your legacy systems to communicate with RAG without a complete overhaul.
📚You may like to read our guide: Embrace Generative AI in 2024: A Detailed Guide for Seamless Team Integration
Challenge 3: Managing Data Quality Across Channels
Poor data quality can cause RAG to generate inaccurate recommendations or operational insights.
Unlike data security, this challenge focuses on ensuring that the information feeding RAG is clean, consistent, and relevant, not just protected.
How to overcome it: To tackle this, retailers need to:
- Implement strong data governance policies.
- Regularly clean, validate, and standardize data from all sources to ensure it’s usable.
- Use automated data-syncing tools to keep your systems up-to-date in real time, preventing discrepancies between different channels.
How RAG Can Be Used in Retail: Key Applications & Use Cases
The capabilities of RAG for retail are as clear as its benefits. But how can it practically be used?
Personalized Marketing Campaigns
RAG allows retailers to craft hyper-targeted marketing campaigns by analyzing real-time customer data—purchases, browsing behavior, and preferences.
This means your marketing is always relevant and timely.
Example: A retailer uses RAG to pull data on recent buying patterns and automatically generates personalized email campaigns with tailored product recommendations, driving up engagement and conversion rates.
Real-Time Inventory Replenishment
Imagine if you never needed to manually track stock again. How much time and resources would that save?
RAG can pull live sales data and inventory updates to predict when products need to be replenished. This keeps shelves stocked with best-sellers and prevents stockouts.
Example: A clothing retailer uses RAG to monitor top-selling items, automatically reordering stock when inventory levels drop below a preset threshold, ensuring popular products are always available.
Customer Service Enhancement
RAG-powered chatbots can instantly access real-time customer data, like order history and preferences, to provide more personalized and accurate responses.
Inaccurate responses can get you into legal battles you don’t want to fight (like when a Canadian airline’s AI chatbot incorrectly quoted a policy–yikes!). But since RAG is real-time and less susceptible to hallucinations you won’t be calling your lawyer anytime soon.
Example: A chatbot powered by RAG helps a customer find a product in their size by retrieving live stock availability across multiple store locations, enhancing the shopping experience.
Trend Forecasting and Merchandising
Want to stay ahead of new trends and anticipate demand?
Retailers can use RAG to pull data from sources like social media, competitor analysis, and market trends to forecast demand and optimize product offerings.
Example: A retailer taps into trending products on social media and adjusts their inventory to match consumer demand, ensuring they’re offering what customers want most.
📚 Want to learn more about LLMs to inspire your use of RAG? Check out: LLM Use Cases: One Large Language Model vs Multiple Models
What Will Your RAG Retail Use Case Be?
Feeling inspired yet? We hope so because it’s time to think up an idea for your RAG application.
You can start by asking yourself (and your teams): Where would more accurate, real-time data have the biggest impact?
Consider areas where decisions rely on large, diverse datasets—such as inventory management, customer personalization, or marketing campaigns.
Then think how proprietary data could be combined with other data sources to give you an advantage over competitors.
For instance, if improving customer engagement is a priority, RAG could enhance your personalized marketing efforts by retrieving up-to-the-minute data on customer preferences and purchase history.
Or, if keeping shelves stocked is a constant challenge, RAG can predict inventory needs based on sales trends and seasonality.
Work closely with your key stakeholders—such as marketing teams, operations staff, and data analysts—to validate and prioritize the areas where RAG could provide the most value.
The Road Ahead: RAG’s Future in Retail
Where, oh where could RAG take retail? Or will retail take RAG…?
We’re excited to see if businesses find a way to merge virtual reality with RAG.
Imagine a personalized shopping experience where customers try on virtual outfits, and RAG retrieves real-time product information and personalized recommendations based on their past purchases and preferences.
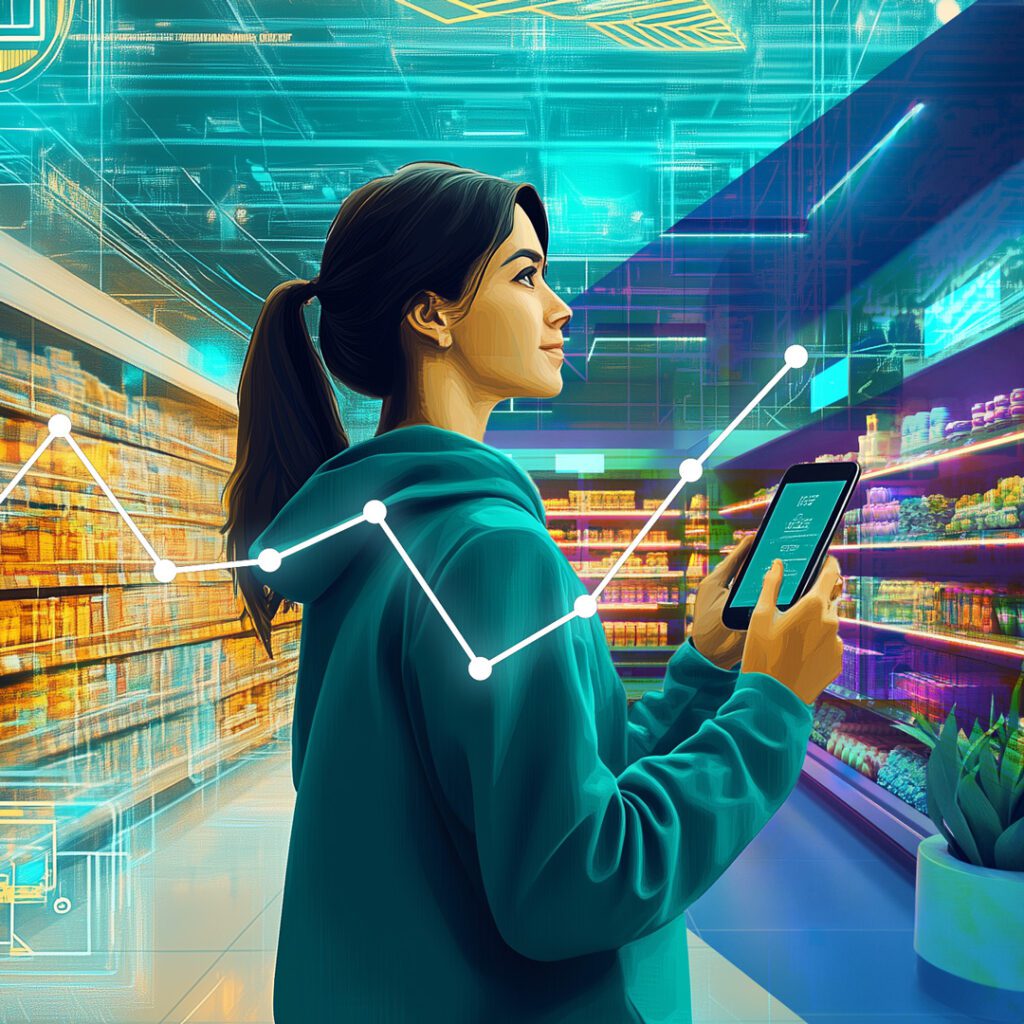
Another area to watch is the combination of RAG with the Internet of Things (IoT) to create smarter retail systems.
RAG could pull data from these IoT devices to optimize stock levels, adjust in-store promotions based on real-time shopper behavior, and even predict which items need restocking before they run out.
Ultimately, the future of retail is about making every interaction more meaningful, and we think RAG will be a key part of that transformation.
Introducing the RAG Accelerator: HatchWorks AI’s Solution to Your RAG Needs
Excited to work RAG into your AI adoption but overwhelmed at the prospect? We get it. It can be complex, time-consuming, and resource-intensive—especially if you don’t know what you’re doing.
That’s why we created the RAG Accelerator. It’s a done-for-you implementation of RAG, allowing you to harness the power of your data without the headaches of development and integration.
HatchWorks AI’s RAG Accelerator offers:
- Customized RAG solutions built around your unique data and needs
- Seamless integration with your existing systems and workflows
- Ongoing support and updates to ensure your RAG system stays cutting-edge
- Full data security and privacy compliance, keeping your sensitive information safe
Visit our service page to learn more about how we can tailor a RAG solution for your organization, or contact us directly to schedule a consultation.
Other AI resources you might like:
- How to Deploy an LLM: More Control, Better Outputs
- How AI as an Operating System is Shaping Our Digital Future
- Embrace Generative AI in 2024: A Detailed Guide for Seamless Team Integration
- RAG: What YOU Need to Know to Apply AI at Work
- The CTO’s Blueprint to Retrieval Augmented Generation (RAG)
- RAG in Financial Services: Use-Cases, Impact, & Solutions
- Harnessing RAG in Healthcare: Use-Cases, Impact, & Solutions
- RAG for Technology: Use-Cases, Impact, & Solutions
- RAG for Communications: Use-Cases, Impact, & Solutions